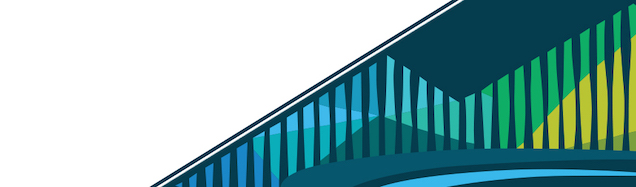
Chapter 4 Intro to Python
Python is a popular programming language that was created by Guido van Rossum and released in 1991.
Python is supported by multiple libraries that support data science tasks:
- NumPy for numerical computing with multidimensional arrays.
- pandas for data manipulation and analysis with data frames.
- Matplotlib for data visualization.
4.1 Main Differences between R and Python
Feature | Python | R |
---|---|---|
Purpose | General-purpose programming language | Statistical programming language |
Suitability | Good at multiple things, including machine learning and deep learning | Very good at statistical analysis but less versatile for other tasks |
Key Libraries | TensorFlow, PyTorch, scikit-learn | Primarily statistical and visualization libraries (not specified in the text) |
Tool for Sharing | Jupyter Notebooks: Open source web application for sharing documents with live Python code, equations, visualizations, and explanations | Same as Python, as Jupyter Notebooks support both Python and R |
4.2 Learning Objectives

4.3 Python Syntax for R Users
An important difference in syntax is 0-based indexing for Python and 1-based indexing for R. This means that in R, indexing starts with 1 and in Python, indexing starts with 0. Coming from R, this means you have to subtract your “R indexes” by 1 to get the correct index in Python.
Other major differences in Python:
4.3.1 Whitespace
Important in Python. In R, expressions are grouped into a code block with {}
. In Python, expressions are grouped by indentation level.
For example, in R, an if statement looks like:
x <- 1
if (x > 0) {
print("x is positive")
} else {
print("x is negative")
}
In Python, the equivalent if statement looks like:
= 1
x
if x > 0:
print("x is positive")
else:
print("x is negative")
4.3.2 Data Structures
There are 4 different data storage formats, or data structures, in Python: lists, tuples, dictionaries, and sets
4.3.2.1 Lists
Python lists are created using brackets []
. You can add elements to the list through the append()
method.
= [1, 2, 3]
x 4) # add 4 to the end of list
x.append(
print("x is", x)
#> x is [1, 2, 3, 4]
You can index into lists with integers using brackets []
, but note that indexing is 0-based.
= [1, 2, 3]
x
0]
x[#> 1
1]
x[#> 2
2]
x[#> 3
Negative numbers count from the end of the list.
= [1, 2, 3]
x
-1]
x[#> 3
-2]
x[#> 2
-3]
x[#> 1
You can slice ranges of lists using the : inside brackets. Note that the slice syntax is not inclusive of the end of the slice range.
= [1, 2, 3, 4, 5, 6]
x 0:2] # get items at index positions 0, 1
x[#> [1, 2]
1:] # get items from index position 1 to the end
x[#> [2, 3, 4, 5, 6]
-2] # get items from beginning up to the 2nd to last.
x[:#> [1, 2, 3, 4]
# get all the items
x[:] #> [1, 2, 3, 4, 5, 6]
4.3.2.2 Tuples
Tuples behave like lists, but are constructed using ()
, instead of []
.
= (1, 2) # tuple of length 2
x type(x)
#> <class 'tuple'>
len(x)
#> 2
x#> (1, 2)
= (1,) # tuple of length 1
x type(x)
#> <class 'tuple'>
len(x)
#> 1
x#> (1,)
= 1, 2 # also a tuple
x type(x)
#> <class 'tuple'>
len(x)
#> 2
= 1, # beware a single trailing comma! This is a tuple!
x type(x)
#> <class 'tuple'>
len(x)
#> 1
4.3.2.3 Dictionaries
Dictionaries are data structures where you can retrieve items by name. They can be created using syntax like {key: value}
.
= {"key1": 1,
d "key2": 2}
"key1"]
d[#> 1
"key3"] = 3
d[
d #> {'key1': 1, 'key2': 2, 'key3': 3}
4.3.2.4 Sets
Sets are used to track unique items, and can be constructed using {val1, val2}
.
= {1, 2, 3}
s
type(s)
#> <class 'set'>
s#> {1, 2, 3}
4.3.3 Iteration with for loops
The for
statement in Python is similar to the for
loop in R. It can be used to iterate over any kind of data structure.
for x in [1, 2, 3]:
print(x)
#> 1
#> 2
#> 3
4.3.4 Functions
Python functions are defined with the def
statement. The syntax for specifying function arguments and default values is very similar to R.
def my_function(name = "World"):
print("Hello", name)
my_function()#> Hello World
"Friend")
my_function(#> Hello Friend
The equivalent R code would be
my_function <- function(name = "World") {
cat("Hello", name, "\n")
}
my_function()
#> Hello World
my_function("Friend")
#> Hello Friend
4.3.5 Importing modules
In R, authors can bundle their code into R packages, and R users can access objects from R packages via library()
or ::
. In Python, authors bundle code into modules, and users access modules using import
.
import numpy
Once loaded, you can access symbols from the module using .
, which is equivalent to ::
in R.
abs(-1) numpy.
There is special syntax for conveniently bounding a module to a symbol upon importing.
import numpy # import
import numpy as np # import and bind to a custom symbol `np`
from numpy import abs # import only `numpy.abs`
from numpy import abs as abs2 # import only `numpy.abs`, bind it to `abs2`
4.3.6 Learning More
If you want to learn more, browse the official documentation for Python.